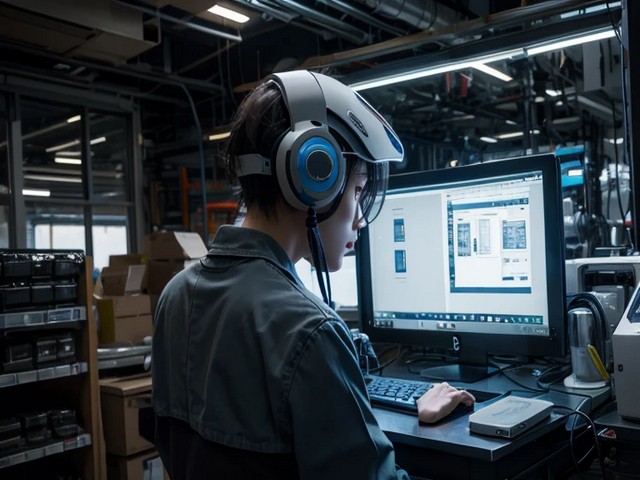
Machine learning is opening up a different, more interesting chapter in human and technological relations – the chapter where machines start to make decisions on their own. This inventiveness of art and science is not only producing sophisticated technology but also paving a gateway for fresher to explore their field. Whether you wish to build a career as a machine learning engineer, or just wish to know more about machine learning and AI, this blog is your key to the future.
The Rise of Intelligent Machines
As the machines are helping them learn better the various industries across the world are going through various changes. From healthcare to finance, the opportunities of the machine learning and AI are actively used for the data analysis, prediction and making the right decision. This revolution is not strictly in a technology space; it requires engineers to work together with creativity to deliver on algorithmic solutions.
Becoming a Machine Learning Engineer
Among the jobs that suit the people who love to combine the tendency of innovative technologies with the practical application of techniques, the machine learning engineer is one of the most interesting. These engineers are on the cutting edge of creating the systems that make it possible for a machine to learn from data. In this blog, we intend to lay out the important attributes as well as knowledge that the candidate will need to qualify for this exiting career choice.
The Importance of Understanding Machine Learning and AI
It’s important to have a layman’s knowledge of machine learning and AI in the modern technological environment. It is crucial to have such a general understanding of the specific technologies as to be able to have an idea of the potential of these technologies, what they can do for you, or what not. Besides, preparing for the future, it informs you of the knowledge that needs to be acquired in order to face the constantly advancing world of automation.
In the next sections we will extend the information about the basic ideas of ML, describe the trends and give the recommendations to those who would like to start with ML. Welcome to the extraordinary process of understanding why and how one of the most fascinating inventions is possible.
What is Machine Learning?
Intelligent computing is a relatively new concept in information technology. It deals with training computing algorithms with specific data to enable them to learn without necessarily having to display human effort. This is a kind of artificial intelligence that brings the capabilities of a system to be able to learn or re-learn itself.
The Concept of Machines Learning
In its essence, machine learning is founded on the theory that machines are learning data. These systems work through channels that allow data feeds to be provided to the algorithms that execute them; this results in the identification of concealed patterns within large quantities of information and the forecasting of outcomes in other scenarios. This process is similar to teaching a student; the more data (or experience) a student is trained with, the more he/she can solve big and complicated problems.
The Role of a Machine Learning Engineer
Indeed, machine learning engineering involves many comprehensive responsibilities to be filled throughout a diverse and complex process.
A machine learning engineer is a person who develops complex algorithms used in machines.
In the case of big data, they are very efficient in converting data into insights as well as training of the model, and retraining of the model. It is one of the most promising scientific areas, as it allows for combining all the skills of software engineering and understanding the intricacies of statistical analysis at the same time.
Machine Learning and AI: A Synergistic Relationship
One should distinguish between ML and AI since these both belong to the computer science field and they are defined in a way that this first one is an application of the second one.
Therefore, one can confidently say that AI is, indeed a rather wide category that encompasses technologies that try to demonstrate intelligent behavior in one way or another; while, at the same time, machine learning can be considered relatively ‘tidy’ since it is defined as the learning capability of machines. Combined they are driving innovation across industries including healthcare, finance, and autonomous systems.
The Difference Between AI and Machine Learning
Machine learning can be defined as a transformative subfield of artificial intelligence or simply AI, and thus anyone who wants to work in this field should differentiate between AI and Machine Learning. Machine learning relates to the creation of models that can help machines learn and act on supplied data. Instead of deciding through a set of instructions based on a list of rules as in conventional programming, machines are learning how to find patterns and draw a conclusion on it all by themselves.
What is Artificial Intelligence?
Artificial intelligence is a large classification of various technologies that help a computer imitate human intelligence to a certain extent. Some of the technologies to include in the big data solution are natural language processing, computer vision, and robotics among others. Thus AI intends to build utilized models in intelligent working, for instance, understanding words, recognizable photos, and so on.
Understanding Machine Learning
While, Machine learning on the other hand deals with the learning power of the machine. They are the process of helping machines learn how they can perform better in the future without being programmed to do so. Machine learning can be considered as being connected with AI because it serves as the foundation for developing most of the types of the latter.
Key Differences Explained
The broader concept of constructing intelligent systems is AI, and the one indicated here is machine learning as one of the approaches towards AI. A machine learning engineer’s primary duty involves designing and optimizing systems through which machines learn from data. This comprises feeding large data into models in a way that the model parameters are adjusted to produce the best outcome.
Practical Applications and Real-World Impact
Today it is hardly possible to name an industry where machine learning and AI are not involved in some or other task or option available. Autonomous cars, identifying what will happen to a patient, and flagging fraudulent transactions are some of the things that machines are now capable of doing. Such applications must be accurate, efficient, and reliable, which makes the machine learning engineer play an important role when creating these models.
Why Machine Learning Matters
Machine learning is rapidly changing the way users and systems communicate, turning conventional systems into smarts that are capable of learning. This mixture of art and science is leading to pre-creation towards forming a future in which machines are capable of thinking, reasoning, and deciding in ways that were hitherto in the realm of human beings only.
The Transformation of Industries
Machine learning is more than hype, it is the new technology revolution with an impact on nearly every industry on the globe. In industries ranging from healthcare to finance, from retail to robotics, the capability to learn is improving both productivity and discovering new possibilities. Machine learning and its subset, artificial intelligence, are the underlying drivers of this shift and allow organizations to tap into the potential of data sourcing the way they used to tap into oil sourcing in the past.
Empowering the Future Workforce
It has now become possible to be a machine learning engineer for the first time in the world. This position plays a key part in the design and implementation of the Machine learning algorithms that underpin all from recommendation databases to self-driving cars. Since AI is rapidly advancing in the capability to perform complicated operations, the need for engineers to build and improve on these systems is on the rise.
Enhancing Everyday Lives
Machine learning is integrated into our day-to-day lives in its ways. Recommendations on streaming services we get, voice assistants that try to recognize our tone and respond to it, and the way predictive text works on our phones are all possible with the help of machine learning and AI. The aforementioned technologies are increasingly becoming integrated into our lives hence making our contact with the technologies natural.
A Collaborative Future
When talking about the combination of machine learning with AI it is possible to note that they bring groundbreaking collaboration between humans and machines. As a result, machine learning systems feed data to patterns and predictions and provide valuable support to professionals in making the right decisions. Here is the cooperation that extends human possibilities, and provides the tools to address existing problems or to look for new opportunities.
How Machines Are Learning: An Overview of Algorithms
Everyday people discover that machine learning is changing the way in which machines learn as well as make decision. For a machine learning engineer or anyone who wants to delve into the field, getting to know what’s behind the algorithms is a good place to start.
Supervised Learning: Teaching Machines Through Examples
Supervised learning is one of the principal types of ML and AI in practice. In this method, data are used which must be previously labeled, according to the names given to the algorithms. This means that through the analyses of the input – output pairs, the system is capable of analyzing new inputs and predicting the correct outputs. It is most commonly applied in classification problems, e.g. differentiating spam emails, as well as in regression problems, e.g. estimating house prices.
Unsupervised Learning: Discovering Patterns in Data
If machines are learning without specific input data for reference then the progress is termed as unsupervised learning. It is used in identification of other hidden patterns or structures from the data in the analysis. It is widely applied to clustering where the data is naturally clustered and association which is used in market basket analysis where the system is expected to notice that certain products are bought together often.
Reinforcement Learning: Learning Through Rewards
Reinforcement learning is one of the special learning categories in machine learning and AI. Here, learning is through operation which consists of action and its consequences being a reward or punishment. This method is particularly useful when there are interconnected decisions necessary in a series including robotics, game playing, and cars mounted with an autopilot.
Deep Learning: Mimicking the Human Brain
Deep neural networks are under the branch of machine learning, and bear the highest sophistication of neural networks. These deep neural networks are meant to mimic the working of human minds and are especially capable when it comes to dealing with excessively large volumes of data. These are critical in applications like image and speech recognition and are the enablers of facets of everyday life, including voice assistants or facial recognition.
Natural Language Processing: Understanding Human Language
An important subfield of machine learning and AI is Natural Language Processing or NLP for short. Natural language processing is the procedure that involves training a machine to understand, process and then produce human language. However, there is a wide array of applications of NLP, hence its uses include chat bots, analysis of sentiments and translation.
The Role of a Machine Learning Engineer
Artificial intelligence is revolutionizing the way society uses technology as the machines are learning from data and then making intelligent decisions. In this process, the roles of machine learning engineers will be right on the front line helping end users translate raw data into knowledge in their organizations.
Understanding Machine Learning
While both refer to a type of variability, these two terms are not interchangeable and hence let’s distinguish between them. Machine learning is a branch of artificial intelligence, which involves using algorithms to sift data; learn from this data, and then decide on its own. Whereas, the term AI is still more general and incorporates, rule-based systems, robotics, and so on. Being a machine learning engineer, you will mostly be involved in developing and optimizing these learning algorithms.
Key Responsibilities
The job of a machine learning engineer has certain duties and it consists of a lot of technical as well as analytical work. You will be responsible for Data Preparation.
Data Preprocessing
A process of preparing the collected data to conduct the next steps of analysis and or model building.
Model Selection
This involves determining whether the best approach to use is supervised or unsupervised learning, semi-learning, or reinforcement learning as a response to the issue in question.
Training and Testing
Employing algorithms and doing trials to set such models in this case.
Evaluation and Optimization
How far is it with the selected model and how does adjusting specific attributes improve on the nature of the model?
Core Skills Required
To excel as a machine learning engineer, you need a solid foundation in several areas.
Programming Languages
Knowledge of programming languages please include Python, R, and Java.
Mathematics and Statistics
familiarity with these notions will be deemed as very good.
Machine Learning Frameworks
Admission requirements require the relevant knowledge of TensorFlow, PyTorch, and other frameworks.
Domain Knowledge
Understanding of the particular area or sector to which you are applying your work.
Collaboration and Continuous Learning
This trend is especially applicable to machine learning and AI, which are continually developing at present. Machine learning engineer interacts with data scientists, software developers, and domain experts in the organization. It is also necessary to accrue knowledge with practice with newer technologies as well as the techniques used within the premises.
Essential Tools and Platforms for Machine Learning
Machine learning has become one of the main foundations of present-day technologies, and it is progressively different enterprises and how machines learn intricate tasks. To become a machine learning engineer one has to familiarize him/herself with certain tools and platforms that render this profession possible and easy.
Integrated Application Development Environments (IDEs)
Choosing an appropriate integrated development environment (IDE) is always vital for the machine learning project. Jupyter Notebook, Pycharm, and Visual Studio are among the Integrated Development Environments that offer flexibility to deal with these problems because they can be used for coding, debugging, or visualizing machine learning algorithms. Any Machine Learning Engineer should find these platforms necessary since they support Python and most other languages used in Machine Learning and AI.
Data Libraries and Frameworks
At the heart of machine learning are libraries and frameworks. Some of the common and moving frameworks are TensorFlow, PyTorch, and Scikit-Learn. For deep learning, TensorFlow and PyTorch are the best models with set APIs, while for traditional machine learning, Scikit-Learn is a perfect fit. These tools help in modeling and make the machine-learning process far easier for machines to learn from the data.
Data Visualization Tools
A major component of today’s approach to machine learning is data presentation, as it helps in its interpretation. To be more precise, Matplotlib, Seaborn, and even Tableau allow machine learning engineers to build interactive data visualizations that identify trends within the data set. Having good data visualization skills helps improve the decision-making process and improves the general comprehension of the learned machine learning models.
Cloud Platforms
Some of the cloud services that are publicly available for fulfilling the needs of machine learning at different scales include Google Cloud AI, AWS, and Microsoft Azure. Modern platforms have many capabilities such as data warehousing and computation, model training and evaluation, and hosting. Cloud platforms can help machine learning engineers use a tremendous amount of computational power in parallel and on demand, which means that they could shorten development times.
Version Control Systems
Code maintenance and management Control are some of the most concise and important obligations in any machine learning work. Some of the common free software collaboration tools include Git and GitHub to write code in groups and to track any code change. With these tools, the secure sharing of sources and methods of machine learning and AI projects can be easily accomplished while the machine learning engineers can easily coordinate their work in teams and handle different versions of the project.
Experiment Tracking Tools
I found out that Experiment tracking tools like MLflow and Weights & Biases are crucial in the Machine Learning model lifecycle. They are used to keep track of experiments, to observe performance, and to maintain model versions. They allow the machine learning engineers who perform these actions to write up results and make their experiments reproducible.
Practical Applications of Machine Learning
Technological advancement is, therefore, being characterized by artificial intelligence; the machines are getting the competence to learn from data and make decisions. Innovations such as these place the machine learning engineer right at the helm steering machines to accomplish what was once considered the impossible.
Healthcare
Artificial intelligence and machine learning are changing the healthcare system to make diagnosis better, treatment individualized, and patient prognosis possible. AI technology is being integrated into diagnosis to determine whether there is an abnormality, quantify diseases or diagnose diseases, and even predict disease outbreaks, improving patient satisfaction and hospital operation.
Finance
In finance, machine learning algorithms are applied to identify fraud, trade automatically, and give recommendations to individual investors. Due to the high volume of data analysis, these systems can locate the patterns and practices difficult to discover for a human being thus minimizing time and risks.
Retail
Machine learning and AI are revolutionizing the retail industry by improving stock management choosing the right products for each client and improving customer care. Customers are being anticipated by machines and even being served by machines through recommendations, and even customer service through a chat box.
Transportation
Within the context of the transportation sector, machine learning has delivered several positive impacts inclusive of self-driving cars, the prognosis of when vehicles require to be serviced, and effective routing. A new generation of machine learning engineers is deploying systems that help machines learn from practical data with improved safety and efficiency of travel.
Entertainment
Machine learning in entertainment involves making recommendations of what content to show the customer, improving the quality of the videos, and generating music and art on its own. You only need to track the user’s attitudes, and over time machines are now able to recommend experiences that capture the audience’s attention.
Challenges and Ethical Considerations in Machine Learning
Machine learning is infiltration in many industries, but this revolutionary kind of technology has its problems and risks. Since machines are learning, they follow and sometimes even extend prejudices that exist in the data used in training of machines. This brought about questions of equal opportunities and discrimination something that the engineers of machine learning need to factor.
Data Bias and Fairness
The basis for the operation of machine learning systems is data, and it happens that they take into account only historical data. If prejudices are presented in the data, then the algorithms may provide some unfair outcomes. Anyone using machine learning and AI has to consider dataset auditing and needs to put methods to avoid bias in place. These improvement solutions are diverse ranging from re-sampling and re-weighting to applying fairness constraints directly.
Transparency and Accountability
I now explore the difficulties associated with each category: Similarly, there is another disadvantage of machine learning models, the complexity of models that are hard to understand. Since these machines are learning, even what they decide might not be easy to explain or understand at some point. These problems introduce new questions of transparency and accountability that reside in this ‘black box.’
Machine learning engineers therefore should aim for explainable AI (XAI) would make models easier to interpret and defend. Trust is not the only benefit of exploitability but-debugging, and adherence to regulatory requirements is an added advantage.
Privacy Concerns
Genetic algorithms and AI algorithms need heaps of data and hence, privacy is a severe problem in such systems. Personal data must be collected and used most carefully and with due consideration to privacy considerations. Such approaches as differential privacy and federated learning allow for preserving user information and, at the same time, facilitate machines to learn. The ML engineers should understand the privacy laws and ethics in this era to safeguard every individual’s rights.
Security Risks
While machines continue to learn and become an essential part of many processes, there is always a risk to their security. Another dangerous class of threats can be called adversarial attacks where some subjects deliberately insert specific inputs to mislead a model. As a result, ML engineers need to come up with strong countermeasures against such attacks, so that the systems are secure and functional. These risks could be managed by the following: Always update models and perform the standard security practices where necessary.
Social and Economic Impacts
The cases discussed above have proved that machine learning and AI technologies entail important social and economic transformations. On the positive side, they contribute to increased rates of utilization and efficiency and, the creation of new employment chances, but on the negative side, they are associated with loss of employment and increase the inequality ’income gap’. It calls upon machine learning engineers and policymakers to target such impacts through the spite of inclusive growth that enhances equitable access to AI perks.
Steps to Start Your Journey in Machine Learning
It’s not hype anymore, but an innovative technology that has the potential to redefine the very concept of how these machines are being “taught”. Begin your journey into this exciting area with these beginning strategies.
1. Understanding the Basics
If one’s goal is to become a good machine learning engineer, then, first, he or she needs to understand the principles of engineering. Get to know what algorithms mean and the ways of data preprocessing, or how to evaluate the model. Some sources include special online courses and basic introductory books that contain more information.
2. Hands-On Practice
Related instruction: Internship is vital in machine learning and AI. Work on problems that force you to build the machine learning models from the ground up. The best approach to find datasets and challenges is through kaggle.com. Just recall that machines are learning best when they’re put into practice.
3. Mastering Programming Languages
Machine learning engineers should be able to code because coding is a basic necessity when it comes to machine learning. These languages come with wonderful libraries and frames worthy of easy application of machine learning algorithms. Success at coding is more attainable when you write code frequently.
4. Exploring Advanced Topics
Once you are aware of each of the models, delve into other broader classes of machine learning and AI. Learn about neural networks, deep learning, and NLP (Natural Language Processing). These areas will lead to opportunities for further challenges and significant assignments.
5. Joining a Community
Joining a machine learning community can shorten some of your learning curve. Join and actively contribute to threads, go to webcasts, and become a member of study groups. The participants express the idea that the possibility to collaborate and exchange information with other like-minded people is one of the networking benefits.
6. Building a Portfolio
Summarize all the work done throughout the methodological complex or in other related coursework by creating a portfolio. Managing a portfolio effectively displays your performance and innovation to the employer. It is important to have at least one or two projects that you have handled before that are best solved using machine learning and AI.
Conclusion
Enough with the hype and FUD around Machine learning, it has bridged what was assumed to be the gap in how machines can think and learn like human beings. Thus, when you start this process, you will quickly realize that it is not an easy task to complete, but the result – machine learning engineer – is well worth it.
Embracing the Complexity
AI and machine learning are difficult and encompassing projects that need scientific methodology and creativity. The beauty is found in the creation of sophisticated algorithms, but the engineering is in the specifications and calculations of these applications. Accept the complexity because real progress is accomplished in the subtle specificities.
Continuous Learning
All in all, it should be understood that the sphere of machine learning and AI is not altogether conservative. The machines however are learning at a faster rate than at any other time and so should you. As a nurse continue to equip yourself with knowledge and skills to enable you to compete effectively. Unlike other conventional learning methods, this kind of learning will not only allow you to catch up with new emerging technologies but also sharpen your innovation skills.
Future Prospects
The future of machine learning and AI is very bright. To be precise, as a machine learning engineer, you will be directly involved with creating it. The work you will produce will impact almost every field that exists, from the health sector to the financial sector, and every other one in between. Opening the door to countless opportunities with the use of UAV and associated technologies, the possibilities are inexhaustible.
Contributing to Society
Finally, remember that ML as well as AI are capable to bringing major shifts in society. Your contributions can help provide a better way of life, address essential needs, define and accomplish goals for humankind. Communication with the dictates of the community, dissemination of the acquired knowledge, and joining hands in order to achieve positive results at work.
FAQ's
What is machine learning?
Machine learning is a subset of AI that is centered on developing models whose ability to learn and improve on experience does not need to be programmed. It is simply the process of feeding data to the computer and using packages to have computers make decisions based on the data.
How do machines learn?
Some of the ways through which machines are learned include; Supervised learning, unsupervised learning as well and reinforcement learning. They consist of presenting data for the algorithms that will allow them to be able to predict, sort information, or recognize patterns so that the machines can learn from the experience of the time.
What skills are required to become a machine learning engineer?
For one to be named a machine learning engineer they have to have adequate knowledge in computer science Mathematics and statistics. Computer programming languages like Python and R and frameworks like TensorFlow and PyTorch are the requirements for this job. Moreover, the knowledge of preprocessing data, model assessment, and optimization respectively also requires a significant role.
What is the connection between machine learning and Artificial Intelligence?
Machine learning and artificial intelligence are somewhat interchangeable terms. Whereas AI is the theory of designing intelligent computing systems that can solve problems in the same way that humans do, machine learning is a sub-corner of this that is concerned with the process of designing algorithms that allow such systems to learn. This relationship makes it possible for knowledge-based AI systems to uncover and seek higher precision in various functions.
How can ML be applied?
Machine learning and its usability are vast including; image recognition and speech recognition, recommendation systems, diagnostics, prediction, self-driving vehicles, and more. It is crucial in many industries because it can handle and interpret big data quickly and correctly.
What are the ethical considerations in machine learning?
Some of the concerns that arise regarding the application of machine learning include; questions of data confidentiality, procedures for eliminating bias in an algorithm, and accountability in explaining results arrived at. To minimize further prejudice in selection and make proper usage of AI technologies, the right and just systems should be set up.